Project Description
Key information
Program title: Data Enhanced Physical Models to reduce material use
Project in the Spotlight: N21022 – P20-22
Funding: NWO Perspectief
Run time: December 2022 – November 2028
Market: Circularity and Material Production
Written by M2i Program Manager: Jeroen van Beeck
DEPMAT Research Program
DEPMAT aims to close the steel lifecycle and to help achieve a fully circular economy for the Dutch steel industry by 2050. The program targets high-quality steel strip used for battery cans and automotive applications. These steels are currently produced using blast furnaces, i.e. a carbon-based iron ore reduction process that produces non-circular steel and has a high environmental impact. DEPMAT’s vision is to ensure that no reduced iron atom is wasted, which can be achieved by re-introducing more scrap as a base-material in steel production. However, the proportion of scrap in high-quality steels is limited by the (uncertain) amount of elements other than iron present in the scrap steel. This causes composition variations in the steel, leading to unwanted property variations. Within DEPMAT, pathways are investigated to improve the control of steel properties by enhancing existing physical material models with data analysis and machine learning techniques. This makes them more quantitatively predictive and faster.
The program develops data-enhanced physical material models that are faster and more accurate than existing purely physical models and that can quickly adapt to varying material compositions. The program also aims to demonstrate the feasibility and benefits of the data-enhanced physical material models through a number of demonstrators. If successfully implemented, DEPMAT’s output will not only increase the proportion of scrap in steel production, making it more circular and reducing its CO2 production, but it will also allow producers and manufacturers to improve product properties by adjusting process settings, producing products within tighter tolerance margins, and specifying steel properties from batch-to-batch or even more locally.
Advanced data methods and machine learning
Within DEPMAT, advanced computational techniques are used to develop hybrid material models integrating physics and data-based approaches. Predictive material models are created for plastic deformation by combining the best of both approaches and accounting for different confidence levels of models and data, while respecting fundamental physical constraints. The program is divided into five work packages (WP), each with its own specific research goals and questions.
In WP1, researchers are developing the scientific backbone for the integration of physics and data-based approaches. The steel microstructure and mechanical behavior are dependent on the complete thermo-mechanical history of the material. However, this history is often not fully available in the dataset. The research questions addressed are: how to fundamentally integrate data in physical models to correct for low-confidence model parts and improve the level of prediction of the constitutive response, and how to formulate the data-modelling part such that fundamental physical constraints are intrinsically obeyed.
WP2 focusses on developing strategies for efficient in-line process measurements to correct model inaccuracies. The aim is to design the most effective interpretation of measurement data to adjust material manufacturing and processing to reduce process variations and to maximize the predictive accuracy of the hybrid models for in-line process control. A probabilistic framework will be employed to aggregate the uncertainty estimates from models and measurements.
In WP3, the researchers develop data-driven methods to establish a direct link between process conditions, composition, and mechanical properties, with close relation to the microstructure. The output of cellular automata simulations is used in crystal plasticity simulations to derive the mechanical properties. Finally, the models that operate on complex microstructures are used to train fast machine learning models efficiently.
WP4 aims to derive a meta model that captures the intermediate and final state of the material in the entire production chain, by intelligently integrating multiscale and multi-fidelity physical and data-based models per process step. The program aims to develop overarching AI process models to capture both temporal and spatial dependencies across each process step, combining physical laws and deep neural networks. Transfer/meta learning frameworks will be used to generalize the AI process models from steel to other metal production processes.
Finally, WP5 focuses on knowledge utilization and application. Within this WP, various knowledge exchange events have been, and will continue to be, organized. Furthermore, near the end of the project, the methods developed in the other WPs will be used to create several demonstrators to show their integrated applicability for steel strip making and deep drawing of metal products.
Overall, the program is thus utilizing cutting-edge computational techniques to develop hybrid models that integrate data and physical models to achieve accurate predictions of the constitutive response and improve the level of prediction of the final product. The program’s power lies in the interactions between the various research topics and close collaboration with the industrial partners.
Collaborative effort
The DEPMAT consortium brings together steel producers and steel users to cover the steel cycle . The steel producers Tata Steel and Outokumpu Nirosta bring in valuable information regarding possible composition variations and knowledge on processing and its effect on the steel behavior. Together, they represent both the low carbon steel strip and stainless steel markets, respectively. Geerts Metaalwaren, Bosch Transmission and SKF bring in their expertise on working with the steels to create products. For them, it is vital that the future steels perform as well, or better, than the current steels they work with, even when the composition varies more due to the increased recycled content.
Eisso Atzema, Tata Steel Nederland, industrial partner
“For Tata Steel, it is great to see the enthusiasm within the research team. The first results are very promising and Tata Steel is following the progress closely with multiple colleagues. Being able to deal with the increased recycled content which will be present in the future “green” steels is a requirement for us to be able to maintain our position as a supplier of high quality steel for the automotive and battery sectors. This program will provide highly valuable insight into how to accomplish this.”
Lloyd Dodsworth, TU Eindhoven, PhD Researcher
“As the main experimentalist in the group of researchers, I regularly interact with the different partners and other researchers within DEPMAT. My project focusses on obtaining information about the effect of various tramp elements on the performance of future green steel. To get the required information and material parameters, I’m applying advanced measurement techniques such as Electron Microscopy and in-situ mechanical testing. From the interactions with the partners, it is clear that the DEPMAT program is very important for the consortium.”
Ton van den Boogaard, UTwente, program leader
“The DEPMAT program is now running at full steam, with a fully staffed research team. After the first startup year, we are now seeing the first promising results of the various research projects. The approach taken in DEPMAT, to investigate the various computing options to enhance the predictions of steel behavior, is working well and there is clear interest from industry on all research projects.”
For more information, visit www.depmat.nl
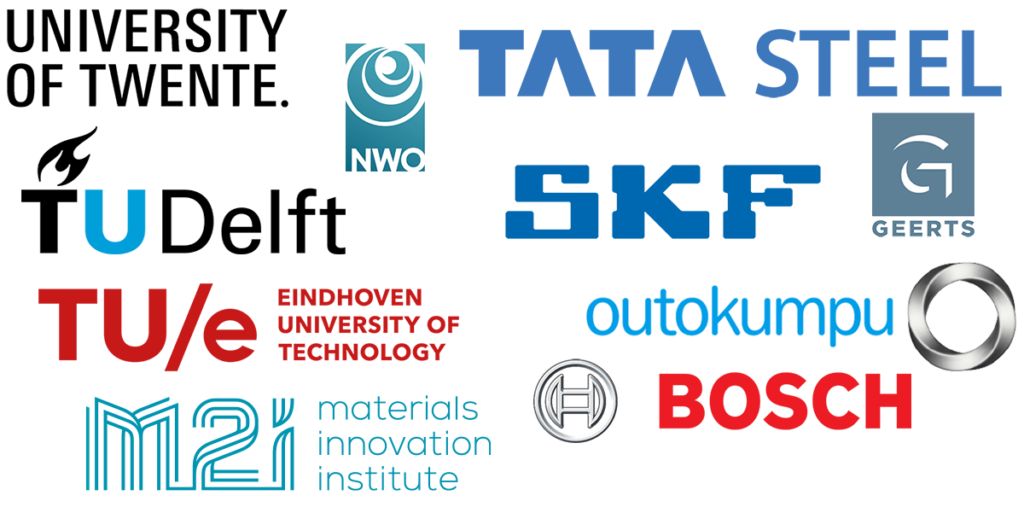